
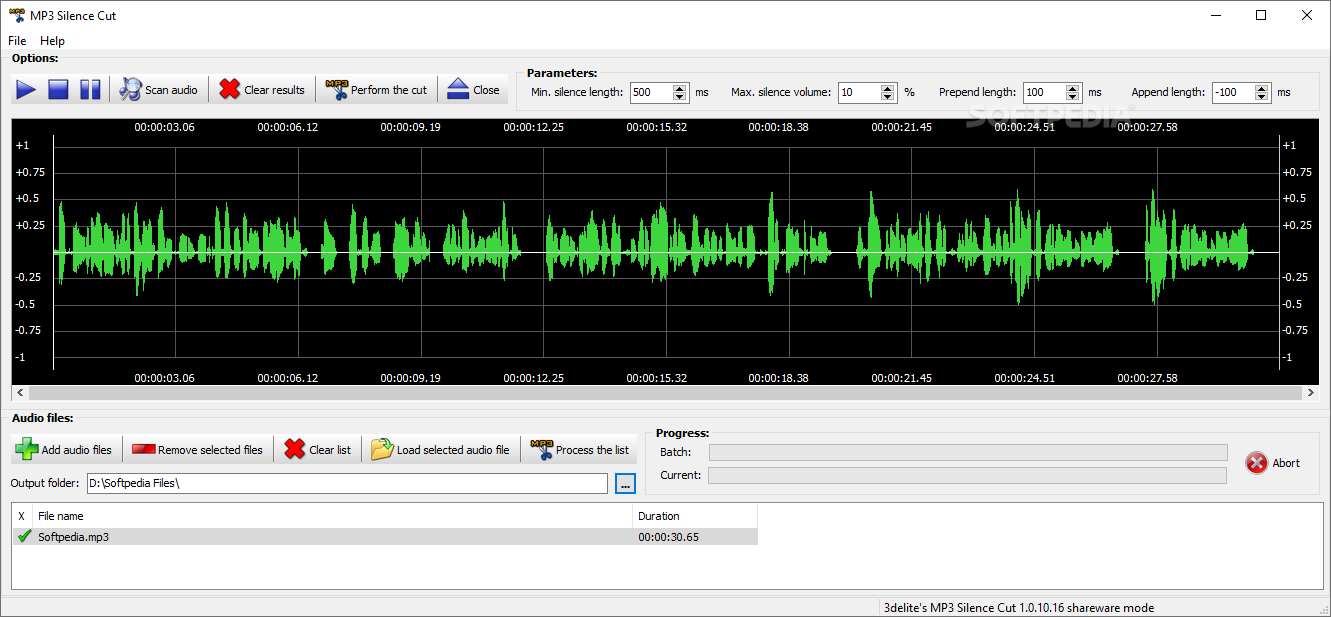
Those deformations and the hyper-parameters used are based on the work of J. To reduce the computation during the training, the data augmentation is not applied to the audio signal but on the magnitude of the power spectrogram. Data Augmentationĭifferent transformations are applied to each training sample to do some data augmentation. Those steps are implemented in train.py and could be changed to test new configurations. Those coefficients are then put on a log scale, normalized over the training set and inputted into the network.
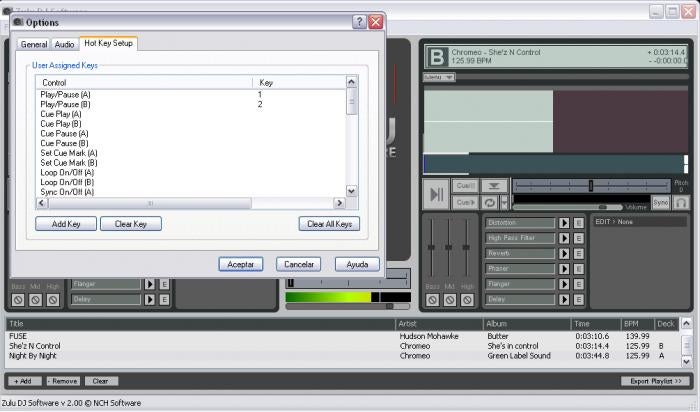
Those steps are done in the file prepare_dataset/prepare_audio.py.ĭuring the learning phase, the spectrograms are loaded, then deformed by the data augmentation and a Mel Filterbank with 80 coefficients between 27. Those matrices are then stored for the learning phase. Then a Short-Time Fourier Transform (STFT) with a Hann window, a frame length of 1024 and Hop size of 512 is applied and only the magnitude of the power spectrogram is kept. Prior to the learning phase, each audio file is resampled to a 22.05kHz mono audio of maximum 1mn30 (the files are split). Then, add the name of the two folders in datasets.json and run the file prepare_dataset/prepare_audio.py to do the processing pre-training of the audio. The files contain the name of the corresponding audio with no extension and the possible files are mixed_train, mixed_val, mixed_test, music_train, music_val, speech_train, speech_val or noise_train. The folder containing the repartition of the data between each set (train, validation or test) for each type of label (speech, music, noise or mixed).The folder containing all the audio files and their corresponding label text files.The dataset has to be separated into two folders: ( HomePage).Įach value is separated by a tabulation. The SoX command line utility is required for the dataset pre-processing.
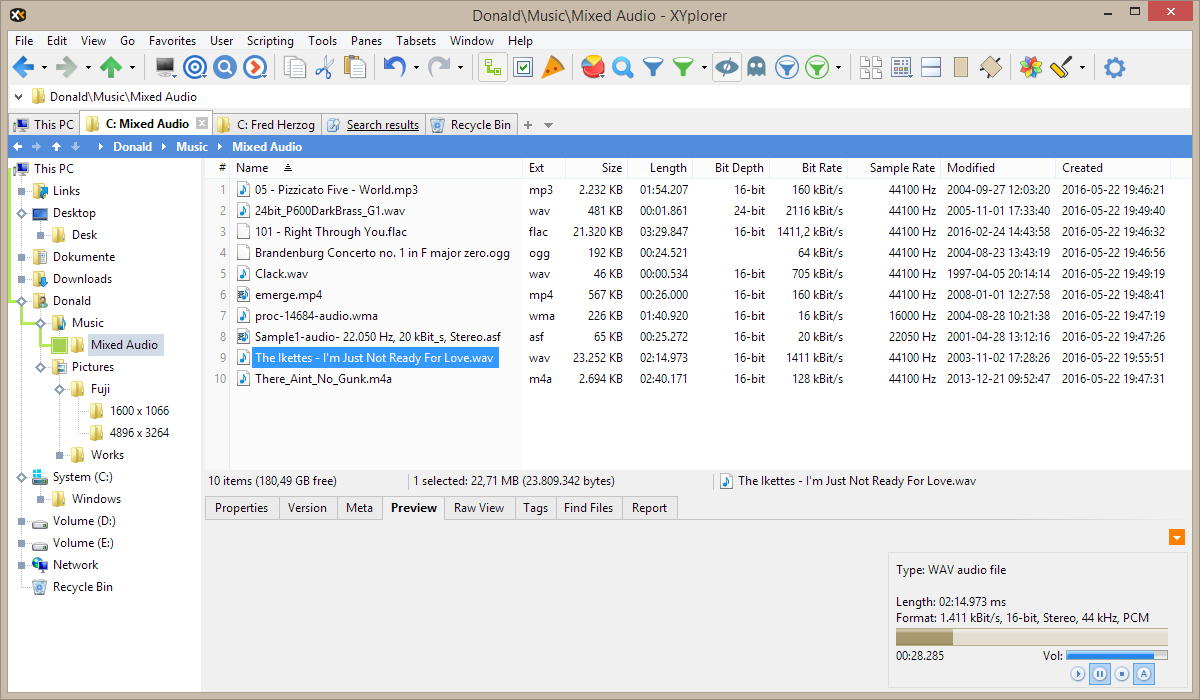
The evaluation implemented in this framework is the same as the one described in this page for comparison purposes.ĭifferent data pre-processing, data augmentation and architectures are already implemented and it is possible to easily add new methods and to train on different datasets. More details about this task can be found in the description page for the MIREX 2018 Speech/Music Detection task. This framework is designed to easily evaluate new models and configurations for the speech and music detection task using neural networks. This repository contains the experiments presented in the paper "Temporal Convolutional Networks for Speech and Music Detection in Radio Broadcast" by Quentin Lemaire and Andre Holzapfel at the 20th International Society for Music Information Retrieval conference (ISMIR 2019). * exp( -(x.^2)/(2*sigma.Python framework for Speech and Music Detection using Keras. Sigma = 0.335 % standard deviation used in Gaussian formula X = linspace( -1, 1, P) % create a vector of P values between -1 and 1 inclusive
AUDIO FILE PEEK DETECTION CODE
This is the code Ive used: = wavread('c scale fast.wav')
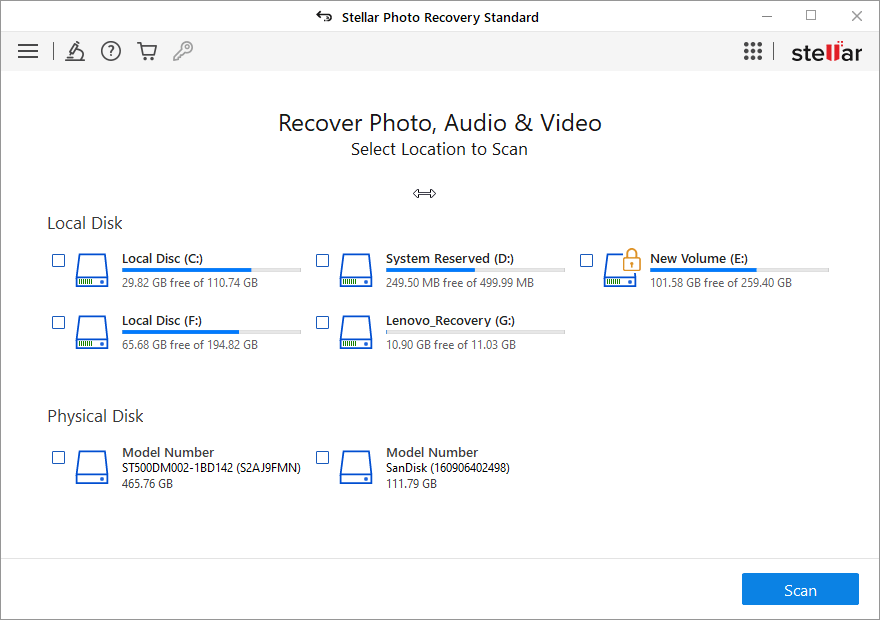
I need to find the x axis points where peaks occur so I can then perform FFT. However I'm still unable to exctract information about peaks. I then came across a peak finding code and merged it with my previous code. I used "findpeaks" but was not successful. I've been trying to find the peaks of an audio signal.
